SRCN Solutions takes a cautious approach to use of AI for reducing maintenance backlogs, emphasising that current AI technologies are not yet capable of replacing the deep asset knowledge and human experience required for accurate and thorough reviews.
Maintenance backlog refers to the amount of unassessed or unendorsed risk that exists within a business.
While AI may eventually offer time savings, SRCN Solutions believes in the importance of using human judgment for complex decisions involving asset health, specific vulnerabilities, and mitigating data quality issues in Computerised Maintenance Management Systems (CMMSs). At SRCN we prioritise ensuring robust assessments over speeding up the process.
Having facilitated numerous backlog and live work reviews over the years, engaging directly with client teams to re-assess and re-prioritise each individual work order, it is a very involved process that typically takes 1-2 days per discipline and there is just no sugarcoating that. This approach provides confidence, and clients are assured that all their live work has been individually reviewed using specific asset knowledge and experience, not just generic rules.
AI Lacks Deep Asset Knowledge
AI systems rely heavily on the quality of the data they are fed. In the context of backlog reviews, much of this data comes from the CMMS,which often lacks the nuance needed for decision-making. For example, AI may not know the difference between a pump that is flagged as a spare but is unreliable versus one that is a dependable backup. This distinction comes from years of experience with the specific asset, knowledge that cannot be easily encoded into algorithms. Similarly, AI may struggle to understand the subtle differences in equipment conditions, such as recognising which valves are prone to sticking or which heat exchangers are under long-term isolation and unlikely to be returned to service.
AI and CMMS Data Quality Issues
A CMMS can often contain incomplete or incorrect entries including:
- Incorrect or ambiguously tagged work orders Work Orders.
- Multiple attempts at repair logged under the same work order resulting in difficulty tracing the true status of an issue.
- Inconsistent data entry, with some tasks documented in full detail and others lacking crucial information.
AI, which relies on structured, high-quality data, is inherently limited by these flaws. In contrast, a human can adjust their review based on this broader perspective, knowledge, and insight.
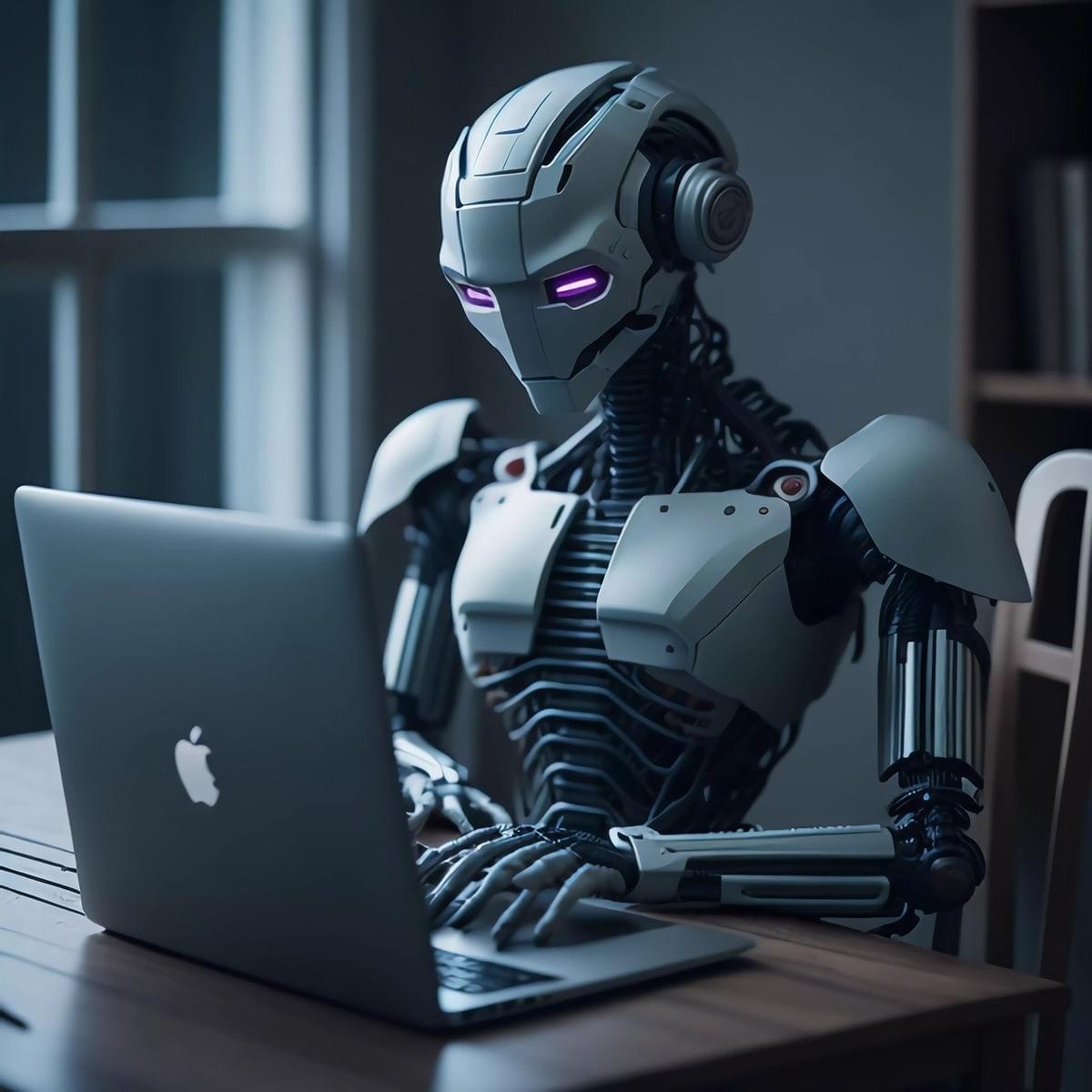
The Value of Human Asset Knowledge
Human engineers, through years of exposure to the operations and equipment, develop an intuition and understanding that allows them to make decisions based on context. AI lacks this capability. It processes data in isolation, unable to account for the subtle factors or historical knowledge that experienced engineers carry in their minds.
The firsthand experience and the asset-specific knowledge of multi-discipline client teams are invaluable in:
- Knowing which pumps are reliable vs. poor spares.
- Understanding which valves tend to malfunction.
- Keeping up to date on projects like replacing obsolete detectors.
- Recognising which equipment is unlikely to return to service despite being in the CMMS.
These teams are also able to mitigate poor CMMS data issues, such as work tagged incorrectly, incomplete orders, work orders with multiple attempts at a repair all against the same order.
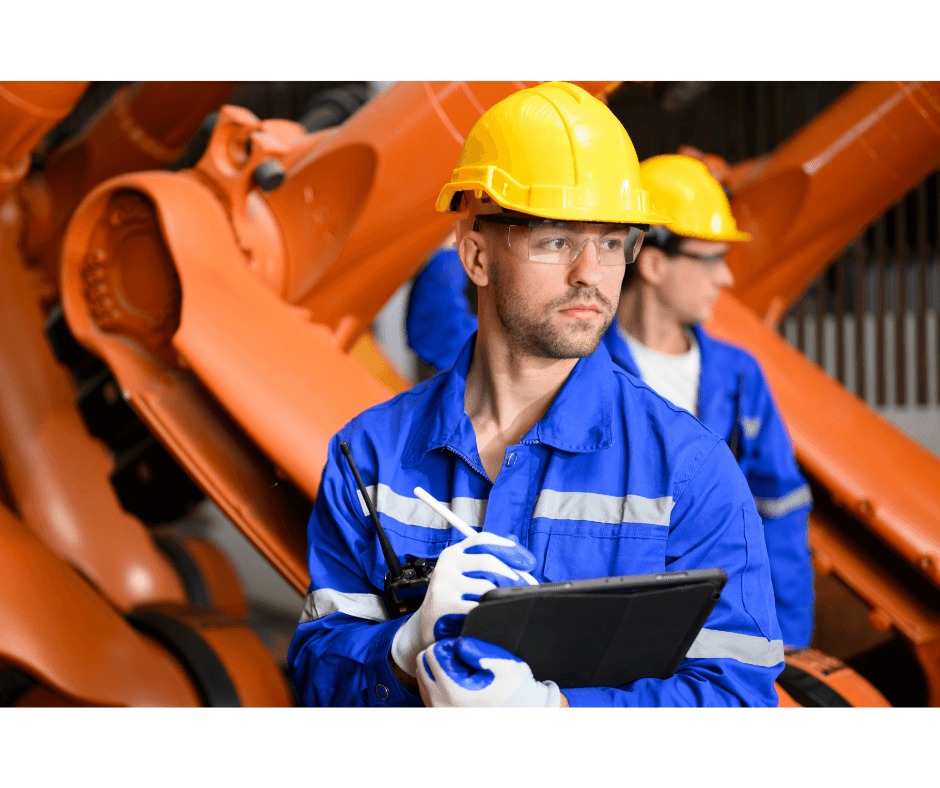
Human Asset Involvement and Ownership, Responsibility & Accountability
Use of AI in activities such as Backlog Reviews could mean asset teams would ultimately miss that deeper understanding of an asset's condition that re-assessing and reprioritising work order by work order brings.
The level of involvement and detailed discussions based on their experience and expertise of these assets brings with it a sense of ownership, responsibility, and accountability that the introduction of AI could dilute and essentially diminish.
Why AI is not the Solution (Yet)!
While AI has its place, the truth is that it cannot fully compete with the deep asset knowledge and human experience needed for effective backlog reviews as the data in most CMMSs is just simply is not good enough.
The potential time savings from AI would come at the cost of:
- Enhanced asset knowledge for engineers.
- Heightened awareness of vulnerabilities.
- Confidence in the robust assessment of risks.
While AI has made significant strides in many areas, its application in backlog and live work reviews is still limited. AI can certainly assist by processing large volumes of data quickly, identifying trends, or
automating repetitive tasks. However, the deep asset-specific knowledge that humans working on these assets possess cannot be replicated by AI, especially when the data AI relies on—typically housed in CMMSs—is often incomplete or inaccurate.
Considering the critical nature of backlog reviews, it is essential to prioritise accuracy and thoroughness over expedience. Until AI technology improves and can effectively handle the intricacies of our backlog review process, we will continue to ensure that our manual reviews are conducted meticulously and comprehensively.
#SRCN/Articles